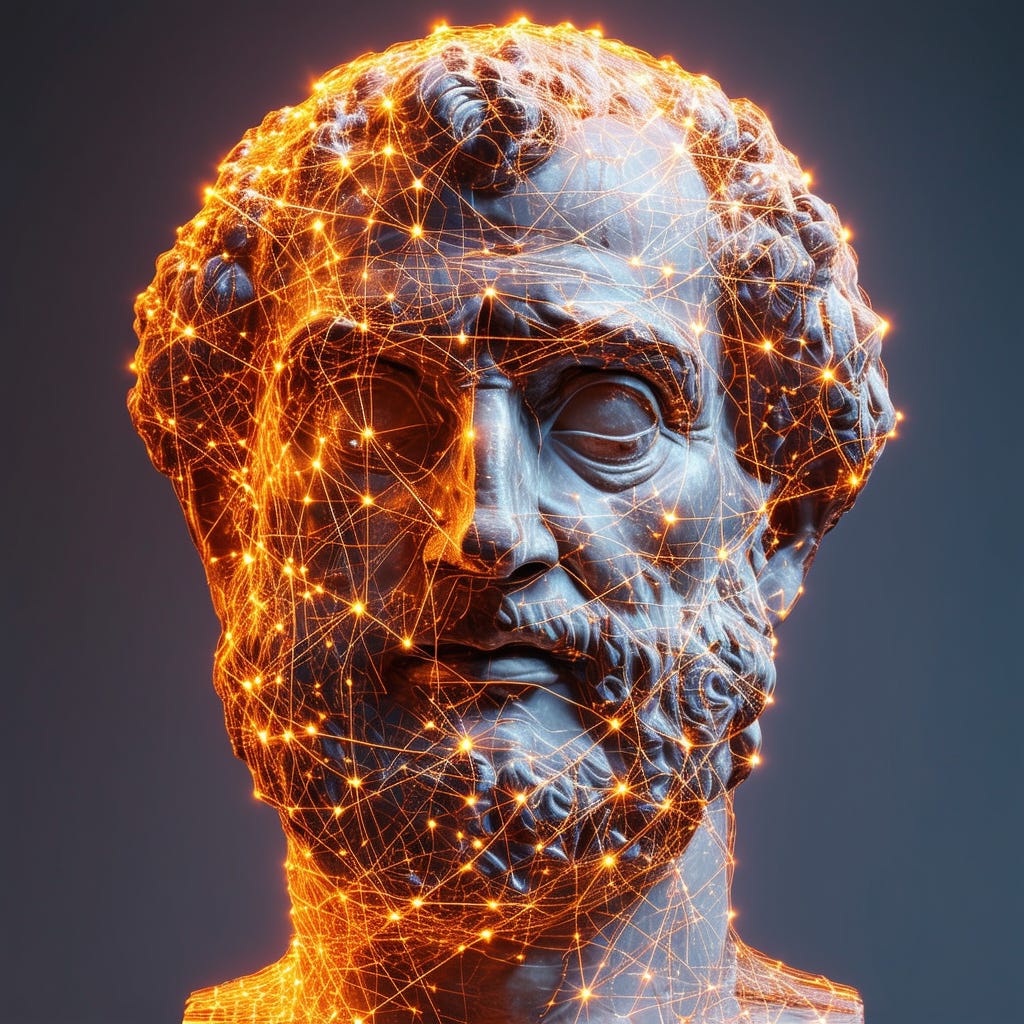
Today, I finally want to explore a concept that's reshaping how I think about writing with artificial intelligence: machine rhetorics. While not completely new, this addresses a challenge many of us face - how to communicate effectively with AI systems.
But before we start, let's take a quick step back. In the classical world, Aristotle defined rhetoric as "the faculty of observing in any given case the available means of persuasion." This may seem simple, but how this definition plays out has changed across the centuries as the “available means” have also changed.
For example, the written word introduced a whole host of available means with the invention of writing … and eventually the printing press, the internet, and even social media.
Think about how we now use punctuation, formatting, and images to communicate and persuade … all available means that did not exist during Aristotle’s time.
The rise of AI brings with it new available means that we might now call machine rhetorics.
Simply put, machine rhetorics is the study of the available means of machine persuasion, that looks beyond prompt design to encompass a broader range of techniques to shape AI responses and behaviors.
Just as traditional rhetoric helps us persuade and communicate with humans, machine rhetorics helps us "communicate" with AI.
As tech writers and content creators, we are at the forefront of human-AI interaction. The way we write, teach, and create content is rapidly changing … not because AI is taking over our jobs, but because our available means of persuasion is widening.
We no longer just have words, images, and video at our disposal, but entire AI systems.
This shift means we're no longer just authors; we're becoming co-authors with machines, and this means understanding how to communicate with AI.
This doesn’t mean the death of rhetoric. Quite the contrary, we are perhaps entering a new golden age of rhetoric.
The Evolution of AI and Rhetoric
Looking at AI as an available means in the field of rhetoric is nothing new. In her 1991 article, Lynette Hunter identified a crucial change in AI technologies that still resonates today.
Hunter observed a shift from AI systems based purely on formal logic towards those that could consider context. This shift is significant for us as tech writers and content developers because it mirrors a fundamental aspect of rhetoric itself.
Built on strict, formal logic, early AI systems operated like complex calculators, following rigid rules without considering the broader context.
Newer AI systems began incorporating context-aware methods, using practical approaches to find adequate solutions when perfect solutions are impractical or impossible … in other words, making good guesses about problems with no strictly definable answers.
That’s one way to define rhetoric … as a method for introducing social and historical context into rational thought to argue or persuade about problems with no clearcut answers.
To illustrate, let's consider a relevant example in our field.
Imagine you're documenting an AI-powered content recommendation system. An early, logic-based AI might recommend articles based solely on exact keyword matches, providing one seemingly inevitable answer. An advanced AI would consider broader factors —the user's reading history, current trends, and even the time of day — to guess at a more relevant and customized response.
As tech writers and content creators, our job has evolved from simply explaining logical processes to helping users understand and interact with these more nuanced, context-aware systems.
This evolution in AI technology brings us closer to the realm of rhetoric. Just as a skilled communicator adapts their message to their audience and situation, modern AI systems adapt their outputs based on various contextual factors.
As early as 2018, Bill Hart-Davidson, a scholar in rhetoric, predicted a significant shift in writing processes. He envisioned a future where most first drafts would be generated by AI, with humans primarily engaging in revision and refinement.
Hart-Davidson suggested that writers would soon use AI-powered software to initiate their work, potentially drawing from the writer's own words or relevant external texts, or what could be called a “knowledge base” … quite similar to how he himself composed his own article … by assembling various notes together into a longer piece.
While acknowledging the growing role of AI in writing, he emphasized that human expertise in revision, curation, and critical thinking would remain crucial, pointing out the need to understand and teach what he called machine rhetoric.
Understanding this shift is crucial as we develop strategies for communicating about and with AI. It's no longer enough to think in terms of strict logical inputs and outputs. We need to consider the broader context in which our AI tools operate.
Rhetoric isn't just about crafting perfect instructions. It's about understanding and shaping the complex, context-dependent dialogue between humans and AI systems.
Defining Machine Rhetorics
To understand machine rhetorics, let's start with a clear definition:
Machine rhetoric is a design practice that allows humans to shape AI technologies with contextual knowledge and understanding, using methods like prompt design, structured content, knowledge graphs, and automation tools.
This definition might seem complex , but we can break it down using a concept many of us are familiar with by now: prompt engineering.
Think back to Aristotle, who developed rhetoric to help analyze speeches after noticing that some were more effective than others. His goal was simple: if we can dissect a speech and understand how it works, we can create better speeches and even teach others to do the same.
Fast forward to today, and we're doing something remarkably similar with AI prompts. Prompt engineering is essentially the art of crafting effective instructions for AI models. It's about breaking apart prompts, analyzing how they work, and learning how to optimize them. In essence, it's a form of rhetoric tailored for machine comprehension.
Let's consider a simple example:
Bad prompt: "Write about AI."
Good prompt: "Explain the impact of AI on content creation for a tech-savvy audience, focusing on recent developments in natural language processing. Use concrete examples and maintain a professional tone."
The second prompt is more effective because it provides context, specifies the audience, narrows the focus, and sets expectations for tone and style. It's a prime example of machine rhetoric in action.
One way to connect prompting to rhetoric might be through the term exordium. In classical rhetoric, the exordium is the introduction of a speech, designed to grab attention and set the tone. Prompt design serves a similar function in machine rhetorics.
Just as a skilled orator carefully crafts their opening to engage the audience and establish context, we design prompts to orient the AI and set the parameters for its response.
We can actually tailor this prompt even more by breaking it apart and expanding it into a structured prompt.
See this lesson in PromptOps for more on structured prompting.
But prompt engineering is just the tip of the iceberg. As we delve deeper, we can learn to analyze and optimize various aspects of AI systems:
Data Curation & Structured Content: Selecting and organizing the information we feed into AI models.
Knowledge Graphs: Mapping out domain knowledge to guide AI processing and make outputs more explainable.
Fine-tuning techniques: Adapting pre-trained models for specific tasks or domains.
By breaking apart these components, we can understand how the best AI systems work and teach each other to create even better ones. This iterative process of analysis, improvement, and education is central to machine rhetorics.
Just as classical rhetoric aimed to make human communication more effective, machine rhetorics seeks to enhance human-AI interaction. It's about making our AI tools more contextually aware, more responsive to nuanced instructions, and ultimately, more useful in our work as writers, educators, and content creators.
Machine Rhetorics in Action
How do machine rhetorics play out in our daily work? Let's explore three key methods, each with a practical example.
Though I will be digging into this further on Cyborgs Writing, I’ll provide small examples of how rhetoric can help us better understand the available means in each example.
Theory can inform practice … but our practice can also inform theory. And the best writers and content developers think about how these two connect.
Structured Content and Knowledge Bases
Structured content involves organizing information in a way that's easily digestible for AI systems. Let's say you're developing a customer service chatbot for a tech company. You might create a knowledge base structured like this:
Product Categories
Smartphones
Features
Common Issues
Troubleshooting Steps
Laptops
Features
Common Issues
Troubleshooting Steps
By organizing information this way, you're helping the AI quickly access and deliver relevant information, improving its ability to assist customers effectively.
In classical rhetoric, topoi (or "commonplaces") were standard categories of argument that speakers could draw upon. Our structured content and knowledge bases serve a similar purpose for AI.
By organizing information into categories and subcategories, we're essentially creating a set of digital topoi that the AI can reference and use to construct its responses.
Mapping Relationships with Knowledge Graphs
Even with structured content, AI systems will only guess at the relationships between pieces of information. Though these may often be good guesses, knowledge graphs are becoming an important tool for introducing ways of thinking to AI models, making outputs more reliable and explainable.
If you don’t know, knowledge graphs are powerful tools in AI and data science that organizes information in a way that both humans and machines can understand.
Imagine a web of interconnected dots, where each dot represents a concept or entity, and the lines between them show how they're related. That's essentially what a knowledge graph is!
Classical rhetoric might help us out here, though.
Aristotle wanted to find a way to organize knowledge in his world. He proposed 10 fundamental categories to classify all things in existence:
Substance
Quantity
Quality
Relation
Place
Time
Position
State
Action
Affection
These categories helped ancient scholars understand and organize the world around them.
Just as Aristotle's categories provided a framework for understanding the world, knowledge graphs provide a framework for organizing and connecting information in the digital age. Here's how:
Substance: Represents the core entities or concepts (e.g., a person, place, or thing).
Quantity & Quality: Attributes or properties of these entities (e.g., age, size, color).
Relation: Connections between entities (e.g., "is a part of," "is related to").
Place & Time: Contextual information (e.g., location, date).
Position, State, Action, Affection: Additional details about the entities' status, activities, and interactions.
Knowledge graphs are a core part of “machine rhetorics,” and we are only just beginning to understand their importance.
Tailoring AI Models with Fine-Tuning
Fine-tuning involves adapting pre-trained AI models for specific tasks or domains. Let's say you're a technical writer for a software company. You might fine-tune a language model on your company's documentation, style guides, and product information. This could involve:
Collecting a dataset of your company's technical documents
Adjusting the model's parameters to better understand your specific terminology and writing style
Training the model on this dataset
The result? An AI assistant that's specifically tailored to write in your company's voice and with deep knowledge of your products.
In classical rhetoric, the concept of kairos emphasizes the importance of adapting one's speech to the specific time, place, and audience. Fine-tuning an AI model is a technological embodiment of this principle.
By training a model on company-specific documentation and style guides, we're essentially teaching it to adapt its "speech" to a particular context and audience, just as a classical orator would.
By understanding and applying these methods of machine rhetorics, you can significantly enhance your interactions with AI tools.
Whether you're crafting prompts, organizing information, mapping knowledge relationships, or fine-tuning models, you're actively shaping how AI understands and responds to your needs.
The Techne of AI Operations
Perhaps the most significant parallel in this overall approach can be summed up with the term techne.
Classical rhetoric was considered a techne - a craft or art that could be studied, practiced, and improved. Machine rhetorics takes a similar approach to AI communication. By breaking down the components of effective AI interaction (prompts, structure, relationships, and adaptation), we're developing a new techne for the digital age.
Just as classical rhetoricians analyzed speeches to understand what made them effective, we analyze AI interactions to improve our communication with these systems.
And just as rhetoric was taught to help people become better communicators, machine rhetorics can be taught to help people become more effective collaborators with AI.
In essence, machine rhetorics is carrying forward the ancient tradition of rhetoric into the age of AI, adapting timeless principles of effective communication to a new technological context.
In future posts, I'll dig deeper into these concepts by exploring how machine rhetorics can be applied in various professional contexts, from content creation, software development, to education.
The field of machine rhetorics is rich with potential, and we've only scratched the surface. As we continue this exploration, I invite you to consider how you might apply these principles in your own work and interactions with AI.
How can you use the art of machine rhetorics to communicate more effectively, to create more powerful tools, and to shape a future where humans and AI collaborate more fully?
By mastering machine rhetorics, we can ensure that we remain the authors of our technological future, crafting AI systems that truly understand and serve our human needs.
Stay tuned for more insights as we continue to unravel the fascinating world of machine rhetorics. The conversation is just beginning, and I look forward to exploring these ideas further with you in the posts to come.